top of page

Ten Days Online FDP on Advanced Remote Sensing and Machine Learning for
Environmental Sustainability
Session XIV : Machine learning: Interpretability & Certification aspects . Dr.R Rajesh ,
Scientist SF, CABS – DRDO, Government of India
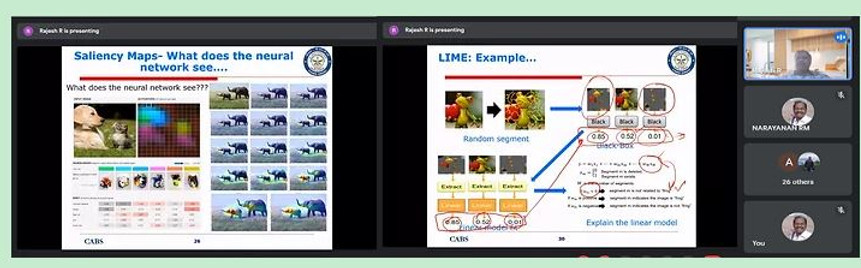
Highlights of the Session - XIV
-
The penetration of machine learning in the fields of gaming, image recognition, speech recognition, natural language processing (NLP), medical field were given an insight.
-
A brief introduction of neural network was given and perceptron, the basic building block of neural network, its architecture was explained. The use of Universal Approximation Theorem in neural networks is briefed.
-
The process of training neural networks along with the steps such as initialising weights, forward propagation and computing loss function; back propagating to update weights, iterating until convergence and the training set, validation set and test set involved in the process were explained.
-
The basics of deep learning involving hierarchical learning, composition of simple non-linear functions, number of hidden layers, specialized architecture of neural networks such as CNN, RNN, GAN, Autoencoder, Attention Models, transfer learning, etc. were described.
-
Few instances, where artificial intelligence failed such as autonomous bus hits para-Olympian in Tokyo, Tesla autopilot hits police vehicles, multiple unsafe and incorrect treatment recommendation in medical field and IPhone X Face ID issue were highlighted.
-
Challenges in this field such as data and model biases, ensuring generalizations, out of distribution inputs and adversarial attacks were explained with the examples such as Amazon hiring problem, rejection of customer loan by Al.
-
The reasons for trust issues on AI such as not having knowledge about what happens in extreme cases, mistakes if occur will be expensive or harmful, etc. were listed out.
-
The factors for interpretability in AI such as showing difference in performances, ability to present a model in understandable terms to human and the pillars of interpretability like faithfulness, plausibility, understandability and stability were described.
-
Correlation graphs, 2D projections, partial dependence plot, residual analysis and how machine learning is used in regulated industries using penalized regression , generalised additive models, quantile regression, piecewise linear models tending to ML one-add interactions, multiple models, surrogate models, local surrogate models like LIME were explained.
-
Interpretability framework involving the inherent and posthoc paradigms, global and local scope of interpretability, model specific and model agnostic application domain and complexity of learned functions, were explained in detail.
-
The frame work of data science involving interpretable machine learning, extraction of relevant information from ML models, PDR (Predictive accuracy, Descriptive accuracy and relevancy), inherent interpretability involving linear regression, decision trees, performance vs. interpretability trade off, etc. were explained in brief.
-
Techniques for improving interpretability of complex models such as feature interaction model of DNN, model distillation , looking inside of feature maps, etc. were described.
-
Simple CNN architecture with low- level features (colours, lines), mid-level features (edges, contours) and high level features (shapes, patterns) was narrated. Saliency maps, heat maps, occlusion maps were given an insight. The local surrogate model, LIME- Locally Interpretable Model Agnostic Explanations was also discussed.
-
AI certification life cycle for aircraft/airborne systems, challenges involved in trustworthiness of AI/ML algorithms and new paradigms like system development assurance, data assurance process, learning model selection and tuning; verification aspects, learning model evaluation, mixability aspects and adaptivity aspects were explained in detail.
Session XV : Estimation of soil Properties using Machine Learning - Dr R.K Setia , Group Leader, Geodata analytics and modeling Group, Punjab Remote Sensing Centre , ISRO, Government of India
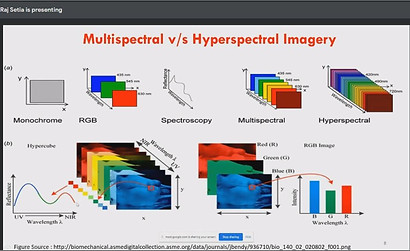
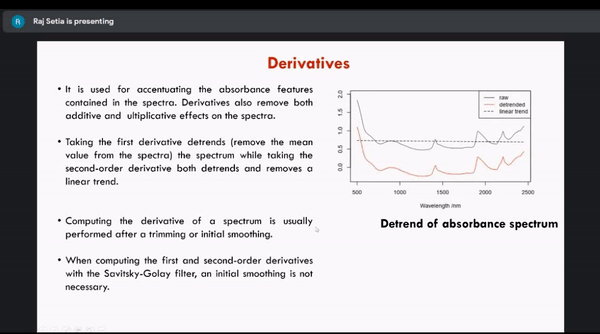

Highlights of the Session - XV
-
Introduction to micro and macro - soil nutrients and its significance in plant growth was presented.
-
The need for Remote Sensing techniques compared to the conventional sampling techniques to predict and quantify soil nutrients was elaborated.
-
The spectral ranges for soil reflectance – Visible, Near IR, Shortwave IR, Mid IR were listed out.
-
Soil spectral behavior followed by microscopic and macroscopic interaction of soil was discussed.
-
Soil texture-sand, silt and clay was detailed. Modelling of soil properties was done with a graphical representation of wavelength versus reflectance. Average spectral reflectance for selected soils of India was given.
-
The concept of Big Data modeling was explained along with first and second order modeling and their difference was discussed.
-
The multiple processing steps such as spectral trimming, moving window average, Savitzky-Golay filter, scatter correction, derivatives and continuum removal were explained.
-
Machine Learning techniques are needed to relate hyperspectral data to soil nutrients, properties and behavior. Supervised learning, Unsupervised Learning was detailed along with the list of common algorithms, Partial Least Square Regression, Support Vector Machine algorithm, PSLR based spectroscopic modeling for AVIRIS hyperspectral data and several other aspects were dealt.
-
The application of ANN in studying the soil properties was detailed in a very elaborate manner.
bottom of page